Orginal Research
2023
December
Volume : 11
Issue : 4
An interesting parameter deduced from confirmed infection number in COVID-19 epidemic
Chow CL, Cheng CH, Chow WK
Pdf Page Numbers :- 267-269
Chow CL1, Cheng CH1 and Chow WK2*
1Department of Architecture and Civil Engineering, City University of Hong Kong, Hong Kong, China
2Department of Building Environment and Energy Engineering, The Hong Kong Polytechnic University, Hong Kong, China
*Corresponding author: Prof. Chow WK, Department of Building Environment and Energy Engineering, The Hong Kong Polytechnic University, Hung Hom, Kowloon, Hong Kong, China. Email: wan-ki.chow@polyu.edu.hk
Received 2 August 2023; Revised 11 September 2023; Accepted 20 September 2023; Published 29 September 2023
Citation: Chow CL, Cheng CH, Chow WK. An interesting parameter deduced from confirmed infection number in COVID-19 epidemic. J Med Sci Res. 2023; 11(4):267-269. DOI: http://dx.doi.org/10.17727/JMSR.2023/11-49
Copyright: © 2023 Chow CL et al. Published by KIMS Foundation and Research Center. This is an open-access article distributed under the terms of the Creative Commons Attribution License, which permits unrestricted use, distribution, and reproduction in any medium, provided the original author and source are credited.
Abstract
In controlling outbreaks of COVID-19, the number of daily confirmed infected cases is useful in deducing parameters to determine emergency management actions such as locking down the cities. However, complicated mathematical models with ordinary differential equations are involved. Resources including manpower and data accessibility are needed. The parameters deduced are always challenged by stakeholders, particularly those from servicing and tourist industry. A simple parameter is proposed to describe the extent of infection by estimating the transient daily infection number divided by the time. The daily infection number in Hong Kong is used to illustrate the approach. This will not require large-scale long-term observation and mathematical modeling or computation.
Keywords: COVID-19; infection number; parameter
Full Text
Introduction
There were difficulties in handling hundred thousands of COVID-19 infected citizens in Hong Kong from 2019 to 2023 [1]. Appropriate control policy [2-9] would protect citizens better, particularly the children and the elderly. Infected patients might need medical treatment but the healthcare system was already overloaded. Tight control is needed otherwise it would affect the normal operation of the healthcare system, as was once encountered in February and March 2022, in the outbreaks in Hong Kong. Close gathering such as catering or entertaining at pubs, and traveling around might lead to rapid spreading of the disease. These activities might have to be tightly controlled, depending the severity of epidemic. Monitoring the infection number and forecasting with simple but useful parameters are thus needed in deciding the appropriate control policy.
A prediction model can be developed from stochastic modeling or deterministic modeling. Physical principles are required with medical information input. The SIR type of models starts from basic assumptions and can be applied only for confirming long-term mass data [10]. The parameters inside have to be adjusted through medical observations. This requires solving a system of ordinary differential equations for the simple SIR model, or the modified versions.
The parameters concerned are complicated, though they can be simplified to describe the possible number of infected persons by one confirmed case. Basic reproduction number and the doubling time of the infection are regarded as important parameters in COVID-19 outbreak, affecting the epidemic wave. Analysis on the epidemic curve employing statistical models to estimate the number of cases [11] might be useful to determine points of major changes in a wave. The process is very complicated and parameters involved have to be updated [12] from a curve fitting exercise, and have to be updated frequently. However, large-scale long-term observations with clinical decisions are required. The process is very complicated and parameters involved come from a curve fitting exercise, and have to be updated frequently. As the situation is so complicated, a simpler approach monitoring the daily I(n) curve (number of infected on the nth day) is more desirable. Emergency management strategy, mitigation, containment or others can be worked out based on this curve.
A parameter is needed to indicate the rise or the fall of infection. The rise tendency in I(n) might indicate possible infection from other countries with huge number of confirmed cases. More stringent inspection procedure to implement at entry points is then needed. The fall trend of I(n) might justify relaxing entry inspection and other gathering restriction schemes.
The 5th wave
Taking the 5th wave [1, 9] in Hong Kong as an example, the daily I(n) curve is plotted using the government data in Figure 1 (taking 1 August 2022 as day 1). It is observed that the situation is severe in the first 50 days. By that time, the vaccination rates among Hong Kong citizens are low. Testing to identify infected cases is another nightmare. Pool tests [13-15] are required to handle huge number of suspicious infections.
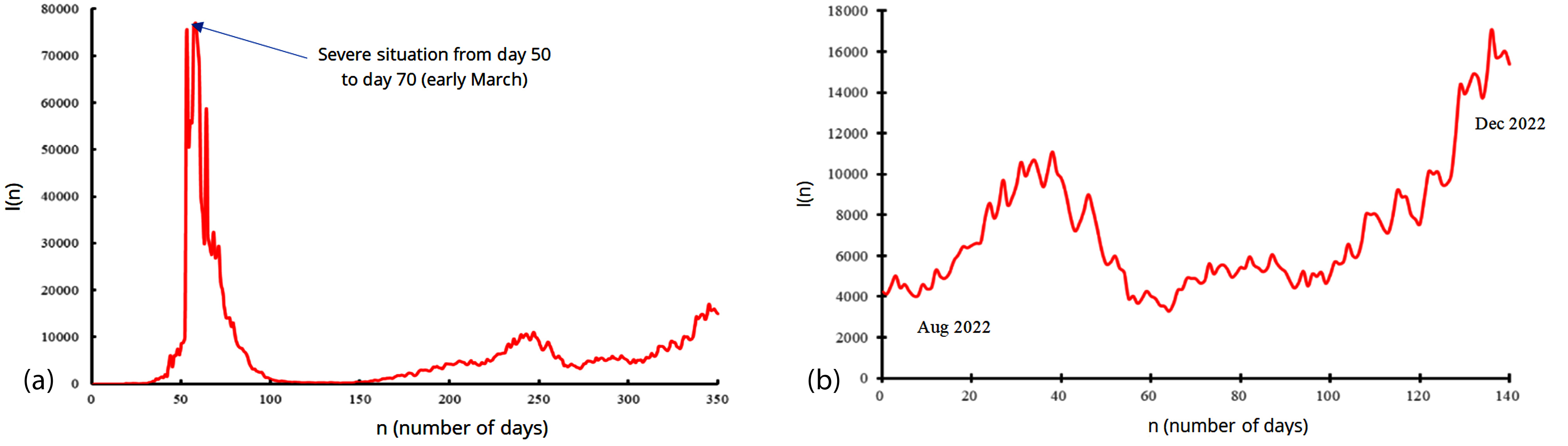
Figure 1: (a) Daily infection number in the 5th wave in Hong Kong. (b) Daily infection since 1 August 2022.
Proposed parameter A parameter Ⅰ(n) is proposed and defined as the ratio of Ⅰ(n) divided by n:
α=(Ⅰ(n))/n (1)
where n is the number of days from the start of the wave, and Ⅰ(n) is the number of infected cases on the nth day. This parameter α shows how fast infection increases, the higher the value, the higher the I(n) and shorter the time to reach it. The transient variation of Ⅰ(n) computed from equation (1) is plotted against n in Figure 2.
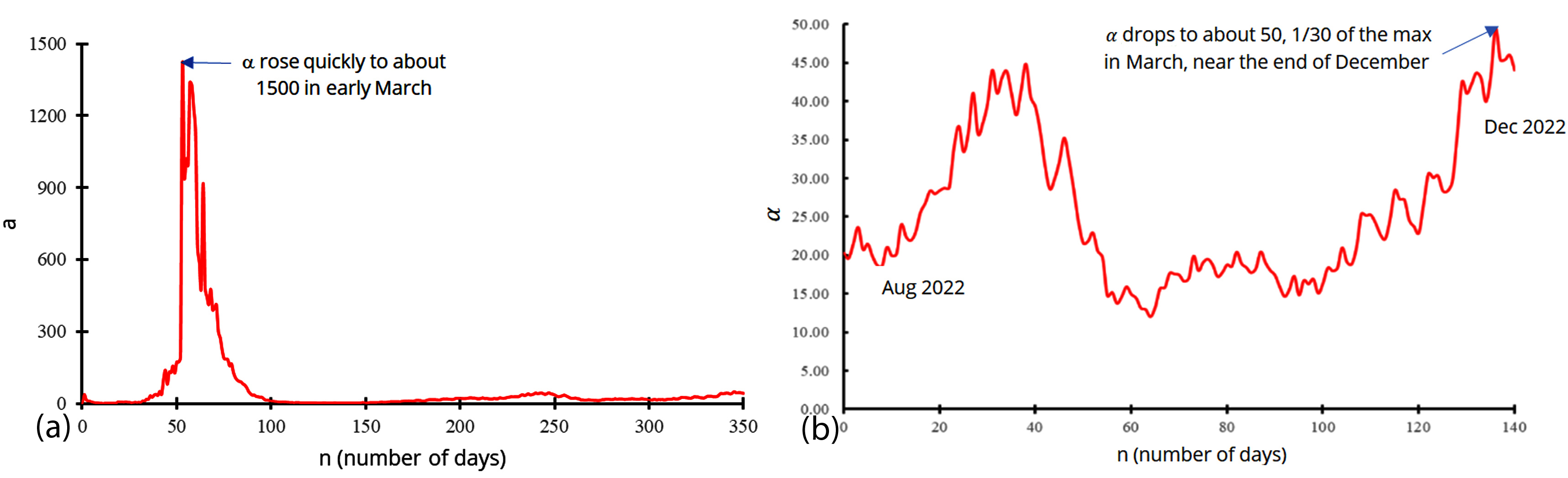
Figure 2: (a) α in the 5th wave in Hong Kong. (b) α since 1 August 2022.
After going through the peak, Ⅰ(n) drops as n increases, giving smaller α. The value of α dropping to an agreed value, say 30% of peak as in above, can give how long it takes the infection number I(n) to drop. The downfall trend of α might justify relaxing entry inspection and other gathering restriction schemes. On the other hand, an increase of α would indicate how fast virus spreads.
Discussion
The Omicron BA.5 subvariant is very infectious [16] and keeping the number Ⅰ(n) high in August 2022. Increase in Ⅰ(n) in August is shown in Fig. 1a, and in α in Figure 2a. The number appears to be large up to the end of December (day 140 in Figure 1(b) and Figure 2(b)), but the Omicron and new variants are not so toxic. Even α is kept high as after August in Fig. 1a, blocking regulations can be relaxed even Ⅰ(n) increased.
The uprising tendency in α might include imported cases from other countries too. More stringent inspection procedure to implement at entry points might be needed if there are new toxic variants.
The simplicity of the present study is a trade-off of the details of the disease spreading dynamics. It does not provide the transient dynamics of the spreading of an infectious disease. Thus, it might not be able to measure the effect of vaccination etc. There are numerous studies which attempted to explain transmission dynamics based on data recorded in the COVID-19 pandemic in some countries. Wu et al. [17] and Tang et al. [18] applied the Susceptible Exposed Infectious Recovered Model (SEIR) to determine the fundamental reproductive number of COVID-19.
Recently Youssef et al. [19] proposed a new statistical model, which is a modified SEIQR (Susceptible-Exposed-Infected-Quarantined-Recovered) pandemic model, to study the dynamics of the spreading of COVID-19. The model is based on rigorous mathematics and the results of the model were verified by COVID-19 data in Saudi Arabia. This proposed model is of great value in monitoring the spreading of an infectious disease like COVID-19.
Conclusions
The parameter α can be applied to study the infection trend using reported data by the government. This is useful to monitor the infection trend, particularly upon relaxation of blocking or inspection regulations to control COVID spread for variants not so toxic.
Conflicts of interest
Authors declare no conflicts of interest.
References
[1] Government of the Hong Kong Special Administration Region. Together, we fight the Virus! Accessed on 28 October 2022 from https://www.coronavirus.gov.hk/chi/index.html
[2] Chow WK, Chow CL. A short note on containment scheme against spreading of novel coronavirus COVID-19. Open J Biophys. 2020; 10:84–87.
[3] Chow WK, Chow CL. COVID-19 and Beyond - A brief introduction to passenger aircraft cabin air quality. ASHRAE J Letter Edit. February 2021; pp.8.
[4] Chow WK, Chow CL, Cheng CH. Virus transmission modes and mitigation strategies. ASHRAE J Letter Edit. July 2021; pp.8–10.
[5] Chow WK, Chow CL. A proposed two-stage quarantine containment scheme against spreading of novel coronavirus (SARS-CoV-2). Indoor Built Environ. 2022; 31:1202–1209.
[6] Chow WK. Ventilation requirements and recommendations for controlling SARS- COV-2 and variants outbreaks in indoor gathering places with close contact. MedRxiv, Posted on 21 June 2022; https://www.medrxiv.org/content/10.1101/2022.06.15.22276447v1
[7] Chow WK. A short note on controlling rapid COVID-19 outbreaks in Hong Kong. Academia. Posted on 16 March 2022; https://www.academia.edu/73873655
[8] Chow WK, Chow CL. Modelling lockdown effects on controlling the spread of COVID-19. medRxiv. Posted on 6 June 2022; https://www.medrxiv.org/content/10.1101/2022.06.04.22275993v1
[9] Chow WK, Chow CL, Cheng CH. Estimation of the total number of infected cases in the 5th wave of COVID-19 in Hong Kong. medRxiv, Posted on 12 August 2022; https://www.medrxiv.org/content/10.1101/2022.08.12.22278708v1
[10] Weiss H. The SIR model and foundations of public health. MATerials MATemàtics, 2013; 13:17.
[11] Küchenhoff H, Günther F, Höhle M, Bender A. Analysis of the early COVID-19 epidemic curve in Germany by regression models with change points. Epidemiol Infect. 2021; 149: E68.
[12] Cheng VCC, Siu GKH, Wong SC, Au AKW, Ng CSF, et al. Complementation of contact tracing by mass testing for successful containment of beta COVID-19 variant (SARS-CoV-2 VOC B.1.351) epidemic in Hong Kong. Lancet Regional Health Western Pacific. 2021; 17:100281.
[13] Chow WK, Chow CL. A discussion on implementing pooling detection tests of novel coronavirus (SARS-CoV-2) for large population. Epidemiol Infect. 2021; 149:E17.
[14] Cheng CH, Chow CL, Chow WK. A discussion on the minimum required number of tests in two common pooling test methods for SARS-CoV-2. Epidemiol Infect. 2021; 149:E179.
[15] Chow CL, Cheng CH, Chow WK. A short note on group testing in coronavirus pandemic. Engineering Archive. Posted on 20 May 2022; https://engrxiv.org/preprint/view/2360
[16] Chaung E, Lam N. Coronavirus: Omicron BA.5 subvariant may trigger new infection wave if it becomes dominant strain, health official warns, as Hong Kong logs 6,389 cases. South China Morning Post, 20 August 2022. Accessed on 20 January 2023 from https://www.scmp.com/news/hong-kong/health-environment/article/3189592/coronavirus-hong-kong-has-room-further-ease
[17] Wu JT, Leung K, Leung GM. Nowcasting and forecasting the potential domestic and international spread of the 2019-nCoV outbreak originating in Wuhan, China: a modelling study. Lancet. 2020; 395:689–697.
[18] Tang B, Wang X, Li Q, Bragazzi NL, Tang S, Xiao Y, et al. Estimation of the transmission risk of the 2019-nCoV and its implication for public health interventions. J Clin Med. 2020; 9:462.
[19] Youssef HM, Alghamdi NA, Ezzat MA, El-Bary AA, Shawky AM. A proposed modified SEIQR epidemic model to analyze the COVID-19 spreading in Saudi Arabia, Alexandria Eng J. 2022; 61:2456–2470.